The Evolution of Conversational AI
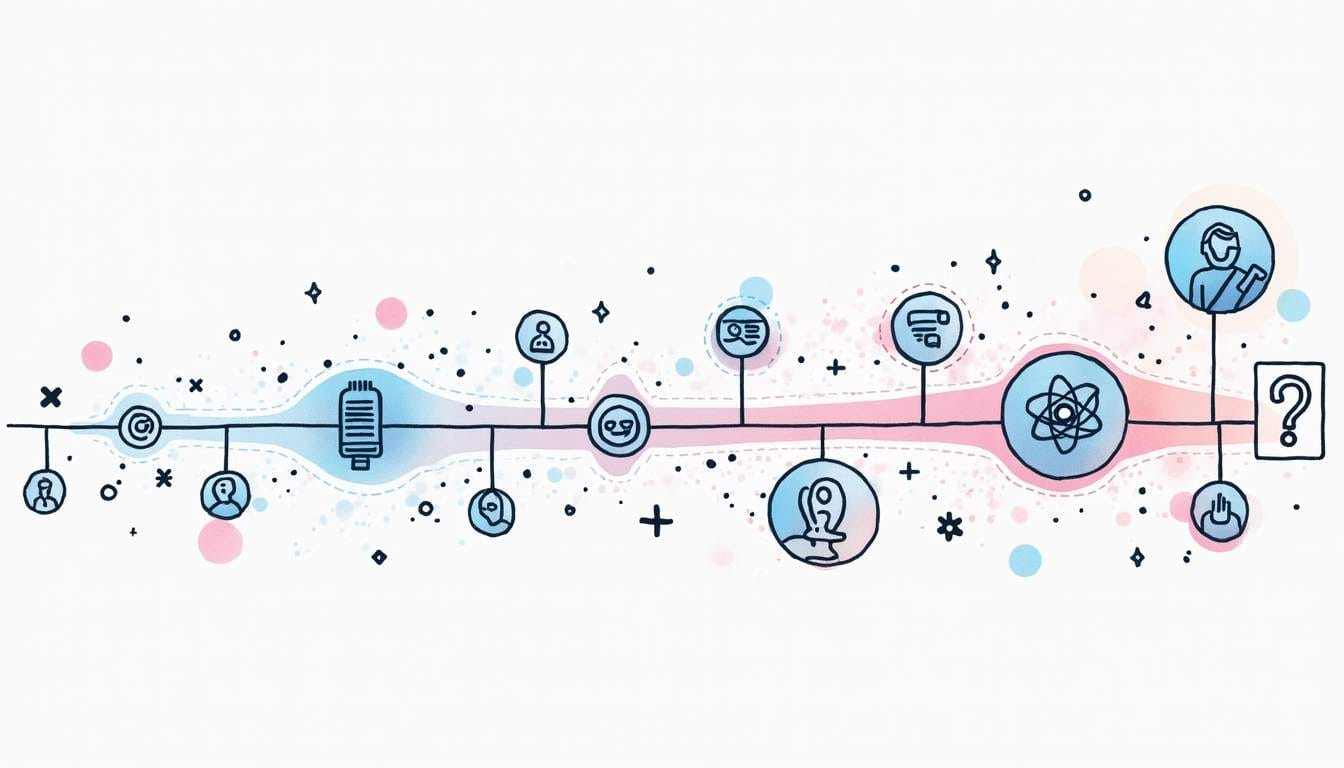
Welcome, curious minds! Buckle up as we embark on an exciting journey through the history of conversational AI, where machines learn to chat, often with an uncanny resemblance to actual humans. From the rudimentary rule-based systems that barely knew how to say "hello" to the cutting-edge GPT models that can compose poetry, code, and cat memes, this tale will tickle your techy taste buds!
Introduction
Conversational AI has come a long way, and we're here to unravel its fascinating progression. Just imagine a chatbot that only responds with “yes” or “no” as opposed to one that can craft entire narratives or discuss the intricacies of quantum physics! The leap from the early systems to today's capabilities is not just a shift; it’s a transformation worthy of a grand narrative.
So, how did we get here? It all started with the basics, and if you’ve ever had a conversation with a stubborn automated response, you might have a few stories to tell about it! But don't fret - we're diving headfirst into the beginnings and beyond!
The early days of conversational AI were marked by rule-based systems that relied heavily on predefined scripts and keyword matching. These systems, while groundbreaking at the time, often led to frustrating user experiences. Imagine trying to book a flight only to be met with a series of rigid prompts that left you feeling like you were talking to a wall. However, this was just the starting point. As technology advanced, so did the algorithms that powered these interactions. The introduction of machine learning allowed AI to learn from past conversations, enabling it to understand context, sentiment, and even humor, which made interactions feel more natural and engaging.
As we moved into the era of deep learning, the capabilities of conversational AI expanded exponentially. Natural Language Processing (NLP) became a critical component, allowing machines to not only understand human language but also to generate responses that were coherent and contextually relevant. This shift opened the door to more sophisticated applications, from virtual assistants that help manage our daily tasks to customer service bots that can handle complex inquiries with ease. The evolution of conversational AI is a testament to human ingenuity, showcasing how technology can bridge the gap between man and machine, creating a seamless dialogue that enhances our everyday lives.
The Beginnings: Rule-Based Systems
Picture it: the late ’60s and ’70s, when the world was grooving to disco and computers were about as chatty as a brick wall. Enter rule-based systems! These rudimentary forms of AI operated on a handful of predefined rules, and while they could play a limited game of “20 Questions,” their conversation skills resembled a toddler learning to speak.
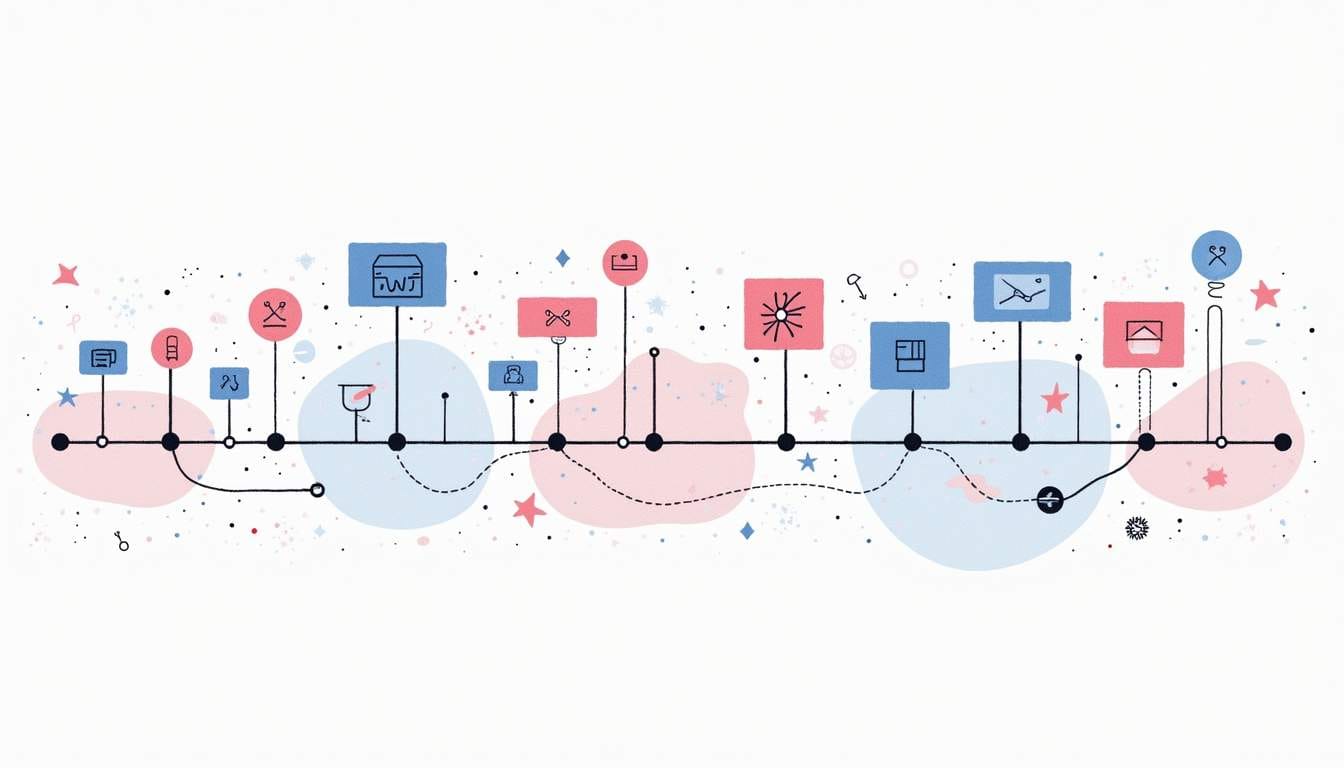
With systems like ELIZA, developed by Joseph Weizenbaum, the foundation was laid. ELIZA acted as a psychotherapist, simply rephrasing user inputs to create the illusion of conversation. Sure, it was a groundbreaking feat for its time, but let’s be real—it was like chatting with an emotionally unavailable friend.
As the years rolled on, more sophisticated rule-based systems emerged, such as MYCIN, which was designed to diagnose bacterial infections and recommend antibiotics. MYCIN was a pioneer, demonstrating that even a limited set of rules could yield valuable insights in specialized domains. It operated on a complex system of if-then statements, showcasing how logic could be applied to medical knowledge. However, despite its impressive capabilities, MYCIN was never implemented in clinical practice due to ethical concerns and the inability to explain its reasoning to human users. This highlighted a critical gap in rule-based systems: while they could process information, they struggled to communicate their thought processes effectively, leaving users in the dark.
Meanwhile, the academic community began to explore the limitations of these systems. Researchers recognized that the rigidity of rule-based approaches often led to brittle performance in real-world scenarios. As the complexity of tasks increased, the systems struggled to adapt. This realization sparked a wave of innovation, paving the way for more flexible models that could learn from data rather than relying solely on predefined rules. The stage was set for the evolution of artificial intelligence, as researchers sought to develop systems that could not only mimic human conversation but also understand and generate language in a more nuanced way.
The Emergence of Statistical Methods
Fast forward to the 1980s and 90s, when the idea of machine learning began to permeate through the world of AI! Enter: the rise of statistical methods. Chatbots started realizing they could flex their computational muscles and learn from data rather than adhering strictly to preset rules.
This exciting shift opened up new pathways. No longer bound by rigid structures, chatbots learned how to interpret phrases based on probabilities. Picture a toddler finally managing to string sentences together; they could make guesses about what you meant. While not perfect, their responses began to feel a tad more coherent (and a little less like talking to a brick wall). Still, there was plenty of room for improvement!
The Rise of Deep Neural Networks and NLP
As we embraced the 21st century, in 2009, the world saw the emergence of something truly revolutionary: neural networks! These gloriously complex structures began mimicking the human brain, firing up the innovation in natural language processing.
These networks, enabled machines to recognize patterns and make connections that were previously unimaginable. As a result, conversational AI had more freedom and creativity. The beauty of neural networks is that they don't just memorize and regurgitate data; they analyze patterns, recognize context, and begin to "understand" language in a way previous systems could only dream of. This opened doors to more lifelike interactions, making chatbots feel less like robots and more like, well, relatable friends—if your friend occasionally switched topics unexpectedly!
The Transformer Revolution
Hold on to your hats, because here comes the Transformer model! Introduced in a 2017 paper entitled “Attention is All You Need,” these nifty models catapulted conversational AI into an era of efficiency and effectiveness. Unlike earlier systems, transformer models prioritize context, honing in on the “attention” wielded by certain words and phrases—essentially learning that context matters more than just rote memory.
This shift meant systems could manage long-range dependencies, understanding how the sentence, "The cat sat on the mat because it was cozy," works. Gone were the days of confusion! The impact was staggering; suddenly, machines began to exhibit a greater grasp of language nuances, quirks, and even idioms. Chatbots found themselves taking on roles that included customer service representatives and virtual friends.
The GPT Era: Dynamic and Context-Aware
Now, dear friends, we arrive at the pièce de résistance: the Generative Pre-trained Transformer, a.k.a. GPT models! If you thought the ride has been exhilarating so far, hold on tight, because this is where the magic really happens.
These models, developed by OpenAI, blew the competition out of the water by harnessing the power of vast amounts of data, training across genres and styles, and learning the nuances of language like never before. Suddenly, chatbots weren’t just echoing sentences; they were crafting essays, providing witty retorts, and even generating stories that a human might find interesting!
The sophistication is astounding. Want a summary of the latest Netflix hit? No problem! Looking for a witty response to your friend's meme? GPT’s got your back! The level of context-awareness has transformed digital conversation, making interactions smoother and more enjoyable than ever.
Challenges and Ethical Considerations
But as we frolic through the fields of chatbot wonder, let’s not forget that where there’s innovation, there’s also a basket full of challenges! For every incredible breakthrough in conversational AI comes a sigh of ethical hues. The capacity for models to generate human-like text raises valid concerns about misinformation, manipulation, and even the potential automation of jobs.
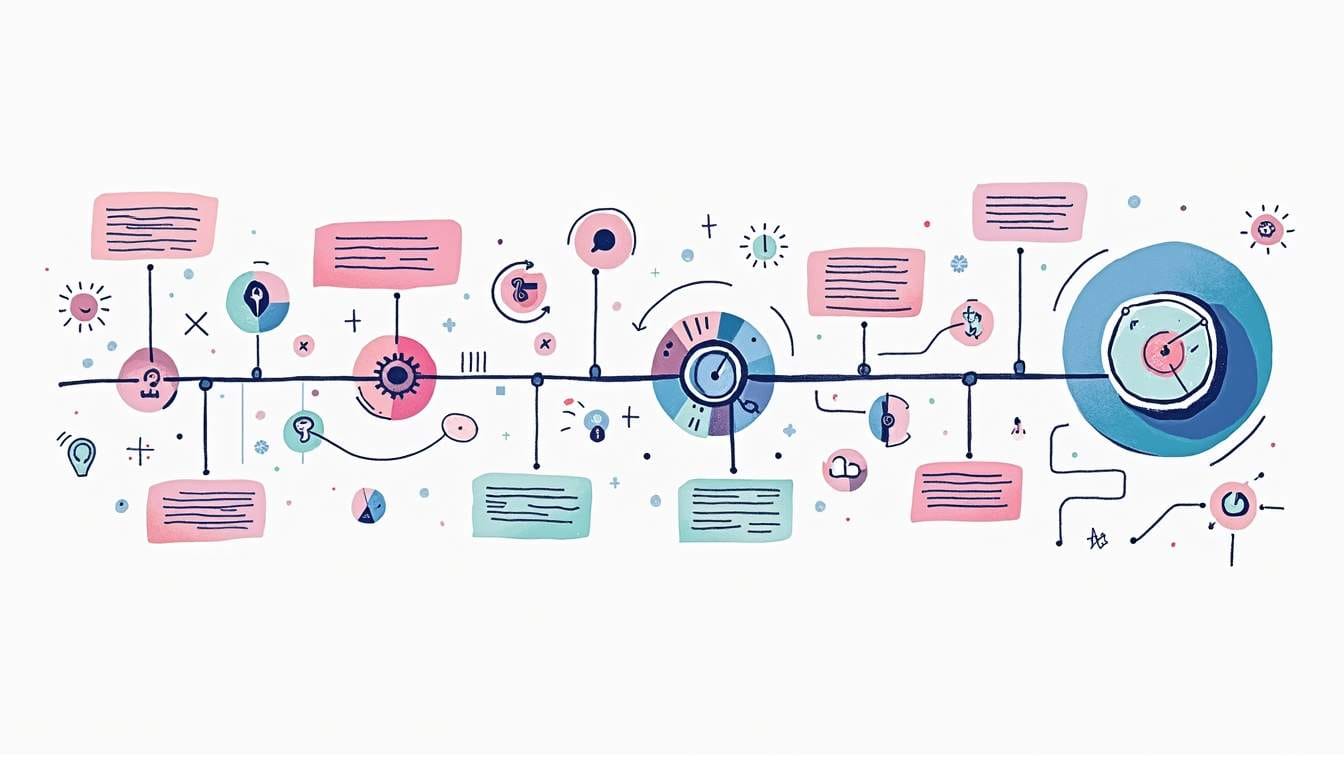
Moreover, bias in AI remains a pervasive challenge. Models trained on flawed data can perpetuate stereotypes or provide skewed results. We can’t have chatbots sounding like they just crawled out from under a rock, can we? Responsible AI development demands checks, balances, and transparency.
Future Directions
Now, let’s gaze into our tech crystal ball and consider where conversational AI is headed next. As the thirst for seamless interactions grows, we are likely to witness further advancements, including better contexts across multiple languages and cultures. Imagine a world where your chatbot understands not just words but the spirit behind them!
The integration of conversational AI with other technologies, such as virtual reality (VR) and augmented reality (AR), will give rise to immersive experiences where AI can engage in realistic environments. Think of interactive narratives that blend storytelling with your own choices—who wouldn't want that?
Conclusion
As we pull together the narrative threads of conversational AI—from its humble beginnings to the splendidly dynamic GPT models we have today—it’s clear that we’re merely scratching the surface of its potential. These conversational companions will continue to evolve, becoming even richer and more integral to our daily lives.
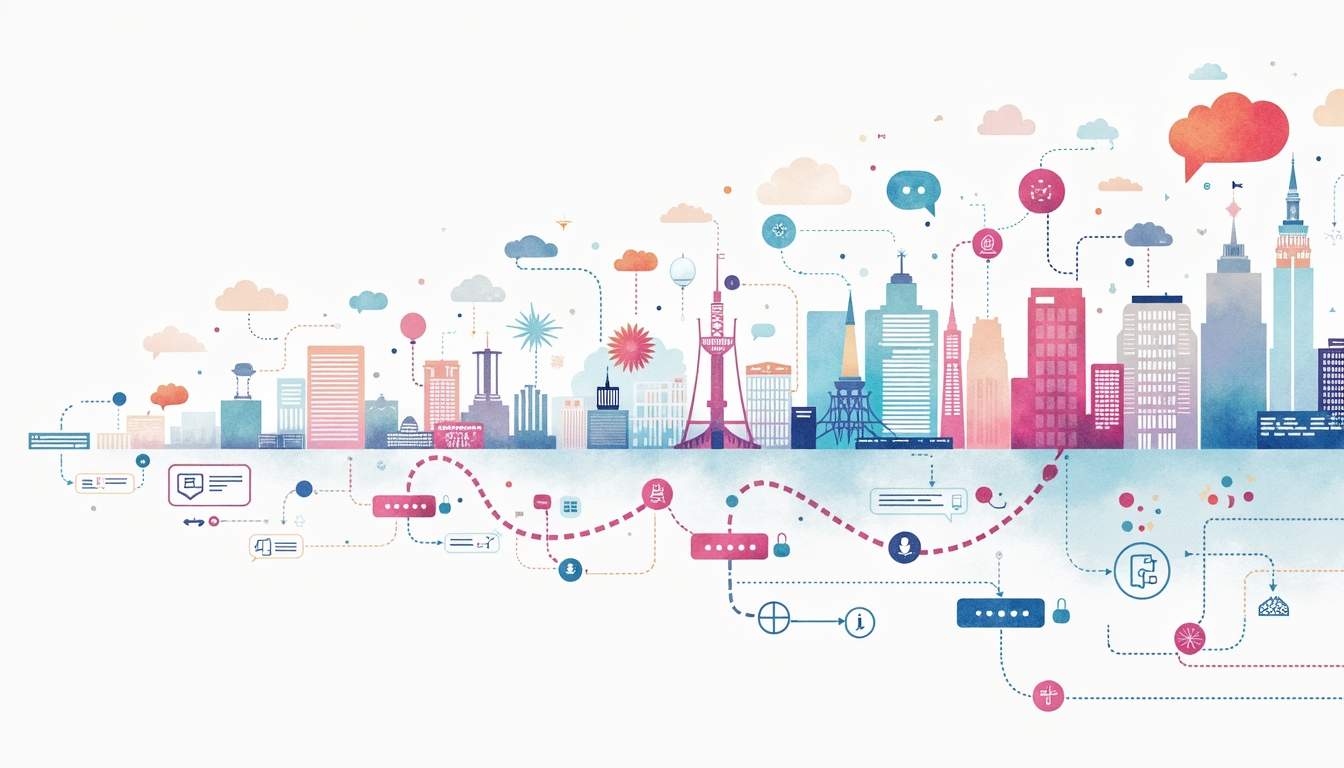
So whether you're chatting with a chatbot about the weather or soliciting advice on cooking techniques, remember: behind that screen lies a fascinating evolution—a history of progress, innovation, and a sprinkle of quirkiness. Here’s to the future, where our digital conversations will only keep getting smarter, funnier, and perhaps a tad more human!
No spam, no sharing to third party.